Harvard Medical School (BIDMC) and Massachusetts Institute of Technology (CSAIL), USA
Authors:Dayong Wang, Aditya Khosla, Rishab Gargeya, Humayun Irshad, and Andrew Beck
Abstract:We adopted the whole-slide standardization algorithm by Ehteshami Bejnordi et al. (IEEE TMI 2016) to standardize all the images to have the same staining color. During training the models, we randomly rotated training patches with 90, 180, or 270 degrees and added extra color noises for data augmentation. Using the new deep learning models based on color-normalized patches, we followed the same framework as our first submission, and embedded the average of two prediction results as the final prediction value. For the second evaluation task, given one whole slide image, we normalized the probability value of each predicted tumor metastases location using the probability values of the first task, which could take advantage of the global information. This method has been updated on November 6, 2016. Instead of generating the likelihood maps with stride 64, the authors generated likelihood maps with stride 4 which led to higher resolution maps that consequently improved their system's performance.
Results:The following figure shows the receiver operating characteristic (ROC) curve of the method.
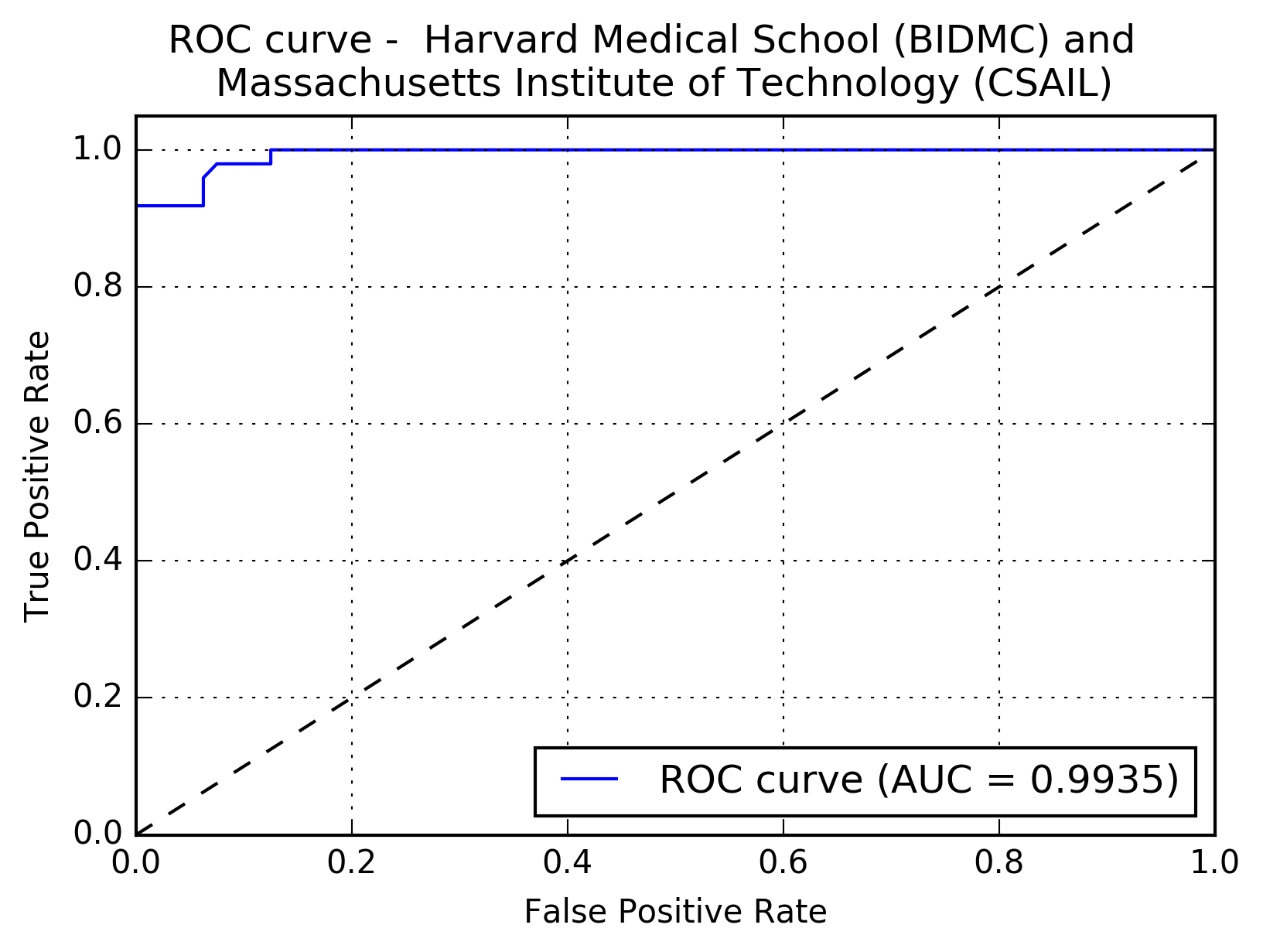
The following figure shows the free-response receiver operating characteristic (FROC) curve of the method.
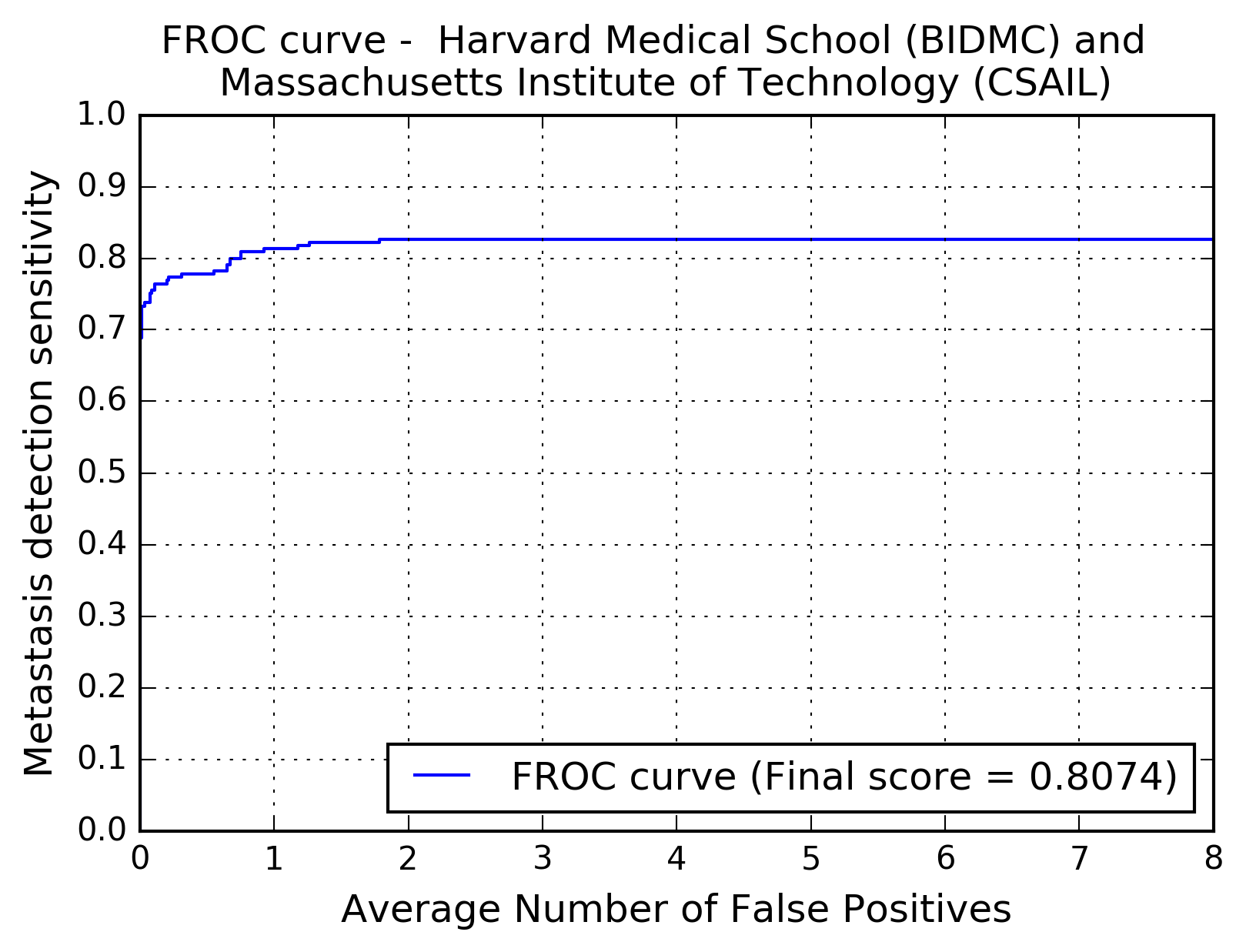
The table below presents the average sensitivity of the developed system at 6 predefined false positive rates: 1/4, 1/2, 1, 2, 4, and 8 FPs per whole slide image.
FPs/WSI | 1/4 | 1/2 | 1 | 2 | 4 | 8 |
Sensitivity | 0.773 | 0.778 | 0.813 | 0.827 | 0.827 | 0.827 |